Language is wide-ranging and diverse, forgot the fact there are hundreds of languages - the way each person uses language represents their locality, education, life experience, generation, social status, gender, beliefs, culture and more that influences intonation, phrasing, word-choices, vocabulary, and accents.
Even if we take English as the prime example of its differences; British, American, Australian, then Global English - the language most noticeable among second-language speakers. Learners own cultural background, social conventions and how they learnt English all heavily influence their understanding of the second-language.
Language by experience
I spent two years teaching English in Brazil (Portuguese speakers), only to return to the UK to teach a Portuguese and the difference was vast, not just cultures, but pronunciation and phrasing. Portugal is also known as one of the European countries with the most English-speakers, yet this particular student had learnt English while working in the UK - my hometown of Bournemouth. He started his life in Britain as a cleaner, who then progressed to management at the council recycling unit - he had a need to improve his English to deal with emails and middle management, his use of English had changed.
Having learnt English at work, he was surrounded by many colleagues who had little education. Their English was used purely for social interaction or for giving orders. As a result, the student lacked the basic knowledge of language structure - he in particular struggled with the Perfect tenses, since many low-skilled native British people rarely use it in its full form particularly in conversation.
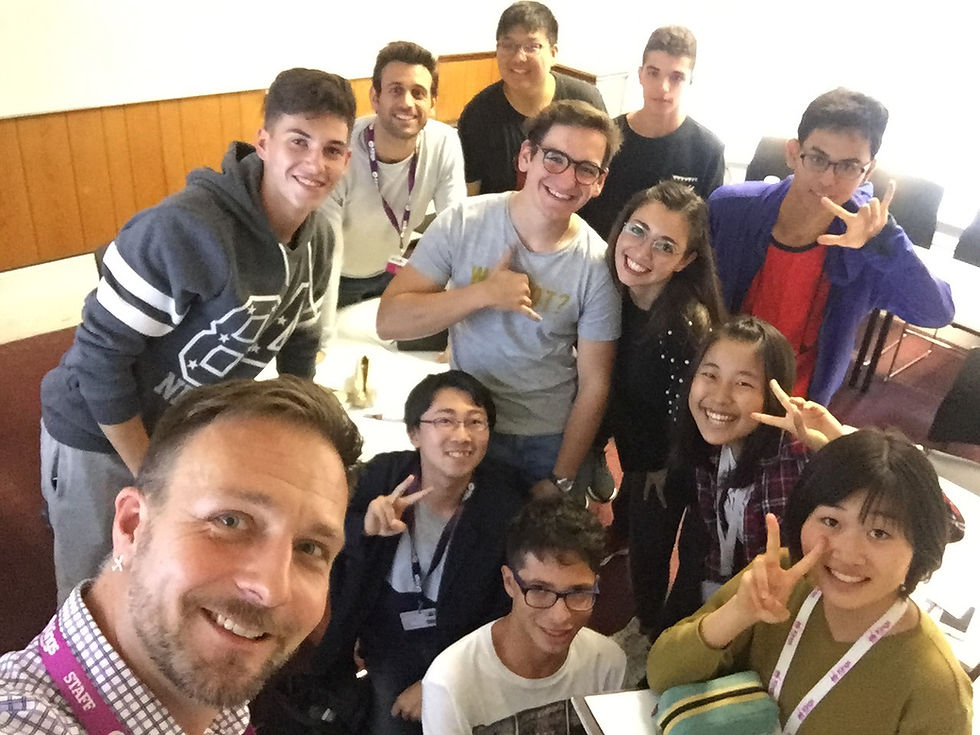
While studying my Post-Grad in teaching I analysed how sentences were constructed, how words could be understood and taught, and most importantly the challenges that language learners have with pronunciation, intonation and phrasing - as well as comprehension. Which leads to our challenge of how we train and prepare new technologies - NLP in particular - to process and act upon many variants of one language.
Language and tech
Chatbots, voice recognition assistants, and other natural language processing (NLP) applications have improved in leaps and bounds. Google’s speech recognition, for example, has a 95% accuracy rate for English words. This is significant because it is also the threshold for human accuracy, meaning Google can match the understanding of the ‘ultimate’ human.
However, the statistics mask several underlying issues with speech recognition which no amount of data might solve.
Language is tricky
Firstly there are some fundamental issues with language itself. A programme might understand every word that has ever existed, but it still won’t necessarily understand the meaning of those words when put together in sentences. One reason for this is that software, no matter how intelligent it is, has no real-life experience from which to understand the concepts behind words. It only has other words.
For example, although we know from our experience what a cat actually is – we have experienced the concept behind the word – a programme can only ever know a definition of the word ‘cat’ based on other words. No amount of data can overcome this problem.
Word collocations, colloquialisms, slang, puns, metaphors, split infinitives and sentences cause problems for language learners, then you need to add in empathy to understand the real meaning behind what is being said.
Everyone uses language differently
Another problem is that every idea can be expressed in an almost infinite number of different ways. This is because different speakers express themselves in their own unique way. Thus, if a programme understands the formal meaning of a sentence like “How are you?” it might not understand the myriad of other common formulations in use (How’s things? What’s up? What’s happening? Etc etc).
Furthermore, if you look at the differences between American and Britain English - if you ask an American if they're 'Alright?' they will think that there is a serious problem. You ask a Brit "How are you?" it feels quite formal and serious. But what about the choice of intonation involved?
Not only can sentences be expressed in a multitude of different forms, the context of the user, their mood, tone of voice, facial expression, body language, emotions, setting, and situation (not to mention the possibility of sarcasm) all add subtle nuances to the meanings of speech which software can’t pick up.
And let's remember when it comes to voice recognition there is the added problem of regional dialects, accents and second language users and this is where issues with accuracy can have huge repercussions, even including discrimination.
Language-based discrimination
While that top line statistic of 95% accuracy sounds impressive, it begs the question – 95% accuracy for who? The answer, it turns out, is white American men. For second language speakers or speakers with less familiar accents the rate is much lower – 78% for Indian English and just 53% for Scottish English for example. And these are all native speakers.
Even more concerning, Google’s speech recognition is 13% more accurate for men than women and 10% lower still for mixed race Americans. This transforms the problem of speech recognition from a technical challenge to a social issue of discrimination based on sex and race. And, importantly, it’s an issue with potentially life-changing implications.
For example what if the speech recognition technology is used for something as important as immigration decisions? Such was the case for an Irish vet who failed a computerised oral English test to stay in Australia, scoring just 74 out of a required 79 for oral fluency. Since English was her native language, we must assume the fault was with the software’s understanding of Irish English rather than thee woman’s ability to speak her own language.
The underlying problem
Racism, sexism and other forms of discrimination based on language are not inherent faults of the algorithms themselves, of course. Rather they stem from the data we feed them. The problem seems to be that these datasets are dominated by white men. For example speech scientists often use TED talks for analysis, but 70% of TED speakers are male.
Despite the obvious progress then, speech recognition and NLP clearly still have a long way to go. As the technology continues to evolve and improve no doubt many of these issues will be overcome. However, it is not only technological advancement that is required. Natural language learning software is only as good as the data it works with. To really improve things we need to improve that data so NLP programmes no longer reflect the in-built discrimination of their makers. This means considering all the challenges related to English, making learning individualised to each user, providing options for the user to select is misunderstood, and allowing users to train devices.
コメント